Abstract
- This paper defines social sensing as individual-level spatiotemporally tagged data and related methodologies. This paper explains the similarity between social sensing data and remote sensing data, and the possibility of combining those datasets
- The major objective of social sensing is to detect socioeconomic characteristics in geographical space, which can thus be viewed as a complement to remote sensing
Introduction
- Uncovering land uses from solely remotely sensed imagery is quite challenging, as socioeconomic features are not directly associated with the spectral reflectance that can be captured by various sensors
- Remote sensors have limited capability to extract socioeconomic attributes and human dynamics such as movements and daily activities
- Several types of geospatial big data are available to capture the spatiotemporal patterns of human activities and thus provide an alternative approach to uncovering land uses and exploring how cities function in a fine temporal resolution
- e.g. taxi trajectories, mobile phone records, social media or networking data, smart card records in public transportation system
- Asserts that different land uses are associated with different temporal rhythms of activities
- Used social sensing to emphasize that geospatial big data can be viewed as an analogues of remote sensing data in social science research
- 기존 연구들은 소셜 센싱 데이터로 land use를 파악하려는 연구 진행해 옴
Social Sensing
- Data set 1) Taxi trajectory in Shanghai, China
- include about 100,000 pick-up points (PUPs) and drop-off points (DOPs)
- Data set 2) check-in records
- spatiotemporally tagged text message posted by a user using a mobile device
- can extract different places such as workplaces, hotels, parks, and restaurants
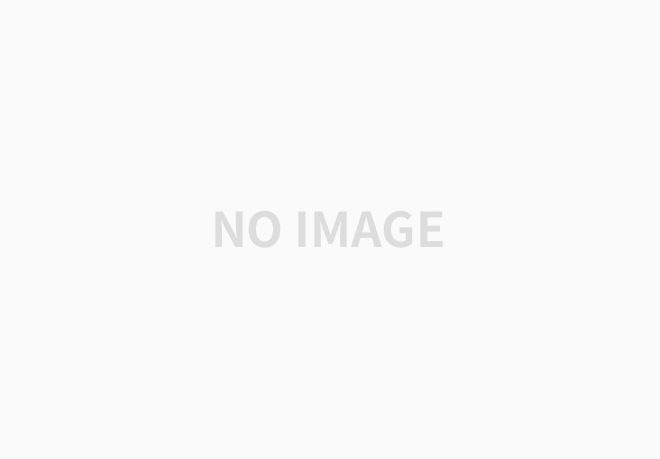
- Compared with the global temporal patterns, given a data source, we are more interested in local temporal patterns because different places (cf. points A and B in Figure 1A) are associated with different temporal signatures
- varying temporal signatures are themselves dependent on the underlying land use features
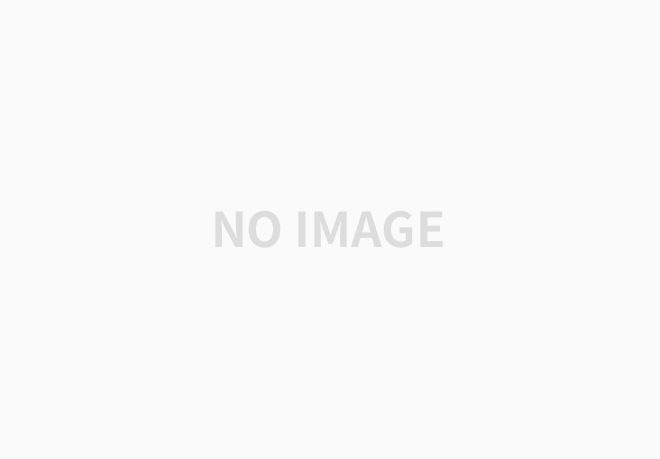
Linking Social Sensing with Remote Sensing
- Social sensing data can be viewed as the analogues of different remote sensing data.
- Social sensing data (Figure 2) show that each place has a different probability of a certain activity
- Social sensing can capture images in various spatial and temporal resolutions, which means that social sensing data are similar to remote sensing data
- Social sensing helps to solve the problem of “inferring land uses from land cover characteristics” in remote sensing applications
- Information extracted from the two different data sources can validate each other to yield more precise results
- 보통 낮은 해상도의 소셜 센싱 데이터를 높은 해상도의 panchromatic 이미지와 결합하여, 해상도를 높일 수 있음
Issues in Using Social Sensing Data ★
- 1) The study area should be rectangular to calculate the temporal variation in human activity
- except for mobile phone data which use the Voronoi polygons generated from the base station
- The resolutions are much lower than those of widely used remote sensing data and it may lead to spatially discontinuous (regarding location) and heterogeneous (regarding land use) results
- → we should choose a coarser resolution to smoothen the activity distributions
- 2) Most social sensing data positively correlate with the population
- In urban fringe areas or rural areas, the activity density is relatively low and thus leads to the problem of small numbers. In such areas, the temporal variations are rather random, and we cannot find a representative pattern after normalization
- As a general rule, the total activity numbers reflect land use intensities and the temporal patterns depend on land use categories (Pei et al., 2014)
- 3) Social sensing data also encounter temporal issues
- 현재 land use classification 연구는 land use와 시간이 비슷하면 similar diurnal patterns of activities를 보인다는 가정을 하고 있음
- The day-to-day changes in trip volume can be attributed to two aspects: special events and long-term dynamics
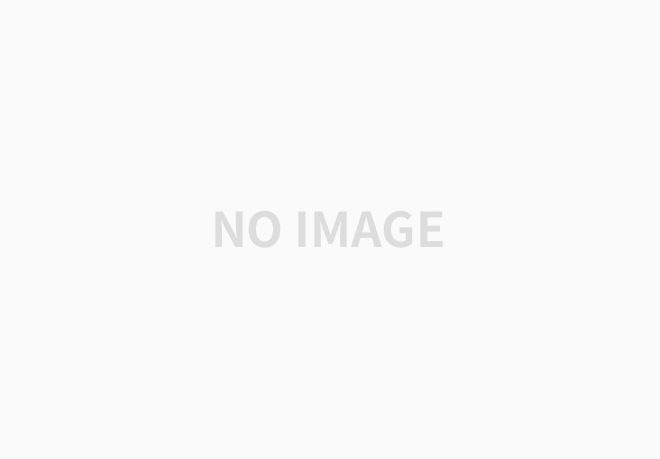
- 4) Most research focuses on a single city rather than intercity
- the diurnal activity variations of various cities are not identical, although some common patterns can be found
- For this reason, unsupervised classification methods are widely preferred over supervised classifiers for social sensing data
- 5) Social sensing cannot directly reveal people's activity
- The observed activities obtained from social sensing data are “proxy activities” such as checking in on social media websites, making phone calls, or boarding a taxi
Beyond Capturing Activities
- social sensing data can be used to extract movements, social ties, and spatial cognition (primarily from social media data) of individuals
Sensing Spatial Interactions
- 소셜 미디어 데이터를 통해 사람들의 활동 및 이동 패턴을 감지하고, 이를 바탕으로 사회적 상호작용과 공간적 관계를 분석하는 방법을 제시
Sensing Place Semantics and Sentiments
- we can not only get the spatial footprints of places from geotagged photos but also can derive human cognitive and hierarchal relationships between places that are captured in such social sensing data
Related Concepts
- i.g. volunteered geographical information (VGI), crowdsourcing geographical information, and urban computing
- VGI includes conventional spatial data such as street lines and POI, which contain little human behavior information and are thus excluded from social sensing data
- In sum, social sensing refers to a category of spatiotemporally tagged big data that provide an observatory for human behavior and the methods and applications based on such big data.
- The major objective of social sensing is to detect socioeconomic characteristics in geographical space, which can thus be viewed as a complement to remote sensing.
728x90
반응형