Introduction
- The increase in social networking sites (SNS) introduced a new opportunity to monitor people's activities and the perception of their surroundings. Location-Based Social Media (LBSM) has been used as a potential resource to characterize social perceptions of places and to model human activities.
- However, LBSM data have various data quality issues such as accuracy, precision, completeness, and representativeness.
- [Research content] This study examines the representativeness issues of LBSM data caused by sampling biases from sociodemographic, spatiotemporal, and semantic perspectives. [Research purpose] The main objective is to provide a framework to examine LBSM-enabled smart city services and their limitations.
Challenges for Social Media-Enabled Smart Cities
- Implementing a smart city requires integrating three components: advanced information and communication technologies (ICTs), open governance, and resident-centered services. A smart city application without paying attention to people would risk disconnecting the service from its users.
- In academia, the discussions about smart cities reflect a broad spectrum of views and researchers recognize the inherent comprehensive characteristics of smart cities.
- Smart city applications must be built on real-time measurements and massive data collection to ensure efficient services. LBSM data are useful due to their uniqueness in recording human experiences and behaviors at fine spatiotemporal resolutions.
- However, Social media data are not universally representative.
The Missing Parts from Social Media Data for Smart Cities
- Researchers have identified five Ws (who, where, when, what, why) and one H (how) in social media studies, and most social media applications focus on the first Ws. This article focuses on the first Ws to demonstrate the representativeness issues of LBSM for smart city applications.
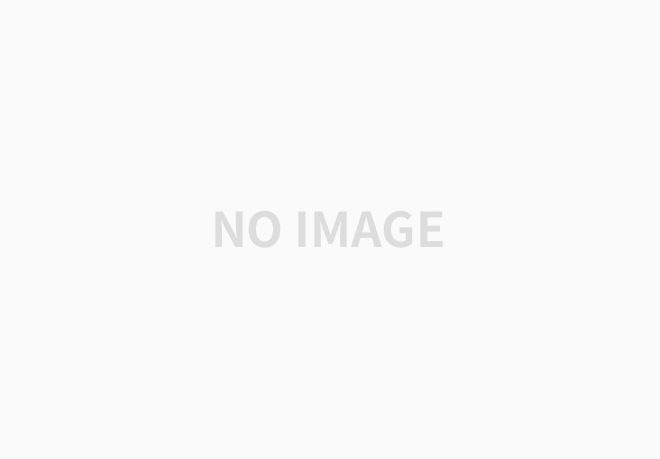
Who Is Reflected by LBSM Data?
- LBSM users are not a random sample in terms of their social, economic, and demographic backgrounds. Recent studies also reported similar findings about the biases of digital trace data and the importance of combining such data with traditional survey data.
- In a case study with Weibo, there was an underrepresented senior population aged sixty-five and older.
- Future studies should conduct a city- or sub-city-level analysis to explore how spatial scales and the modifiable areal unit problem (MAUP) could affect the results.
- Adopting stratified sampling or combining LBSM samples with other public survey data would help mitigate the influence of LBSM user sampling biases.
Where and When Are Things Happening in LBSM?
- There are inherent biases and representativeness issues in the spatiotemporal data acquired from LBSM.
- case study 1) Some locations tended to be oversampled in LBSM data in Austin, Texas.
- case study 2) There was a clear time lag between Bluetooth data (real-time) and LBSM data (not that real-time).
- case study 3) One should be careful using LBSM, especially for emergency planning and disaster management because of spatial errors in geotagged posts.
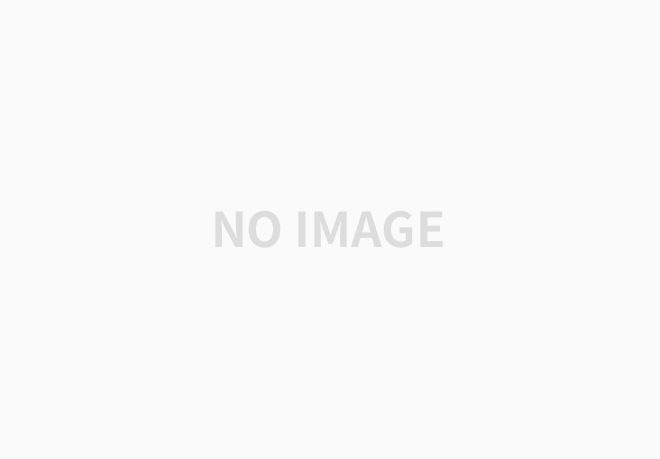
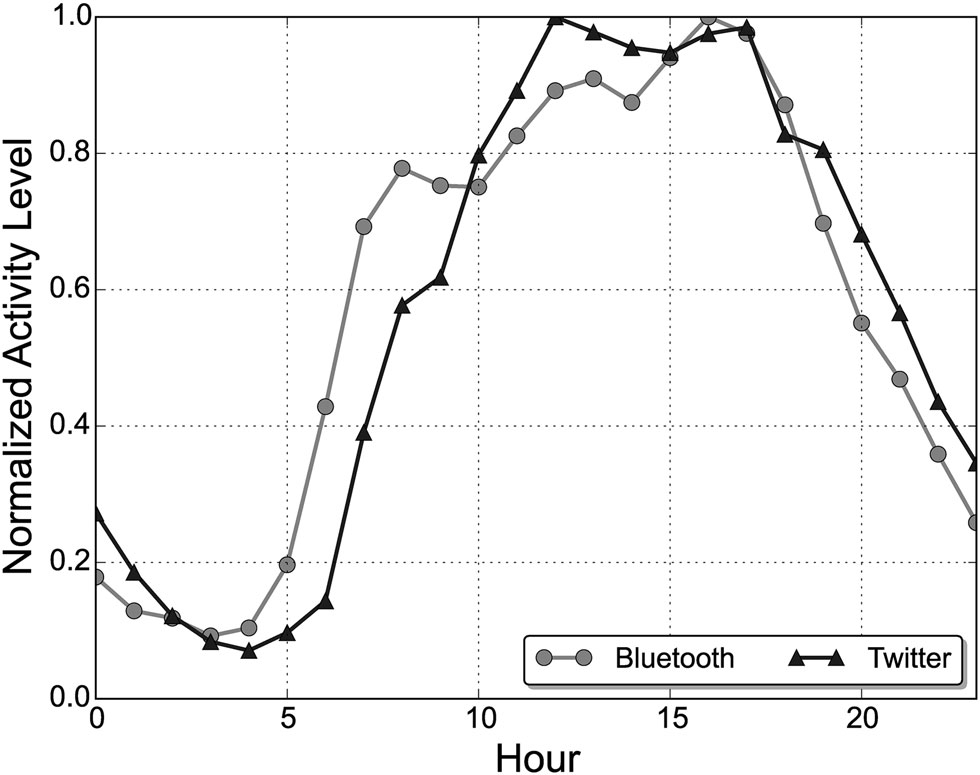
What Do People Talk about on LBSM?
- Social media users are more willing to publicly discuss topics related to their personal life rather than traffic, politics, or urban planning.
- case study 1) Top ten verbs are extracted from Weibo using fuzzy c-means. Social media users usually prefer to share topics related to their daily lives.
- case study 2) Verified public accounts rarely posted on Twitter. This lack of discussion brings challenges to the application of LBSM data to smart city services that require topic extractions.
The fuzzy c-means algorithm generates a membership degree representing how well each verb fits into a certain category. The final results in each category include verbs with a membership degree larger than 0.8.
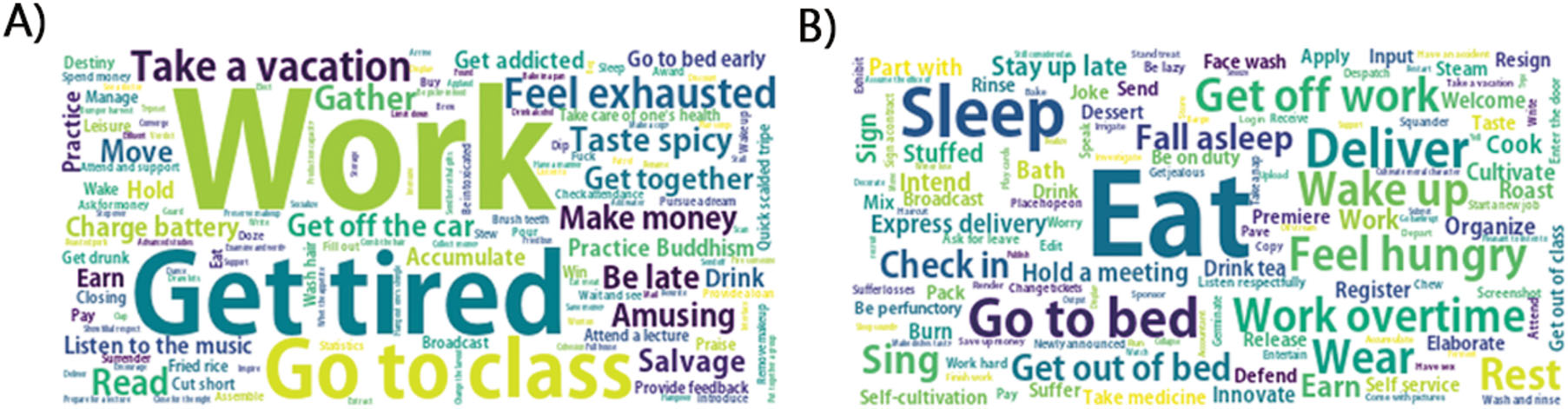
Discussion and Conclusion
- Not fully discussed challenges in this article:
- Other data quality issues of LBSM: ① only 1% of geo-tagged data can be obtained using application program interfaces (API) ② fake check-ins and bots ③ most demographic profiles on LBSM are estimated by algorithms or self-reported making it difficult to validate ④ LBSM data are biased toward overrepresenting "central users" ⑤ considering how LBSM data biases evolve is important
- Distinctions between smart city services: every smart city service has its objectives and functionalities leading to different data needs
- The four Ws are often inseparable. Various factors including user, location and time, and type of activities affect LBSM data quality.
- There are several ways to mitigate the potential problem.
- always be supplemented or corroborated by other data sources
- identify target user group from SNS data
- due to the low spatiotemporal sampling resolution of LBSM data, it is necessary to reevaluate the validity of classic mobility models, measurements, and algorithms